Using machine learning to control stock record inaccuracies
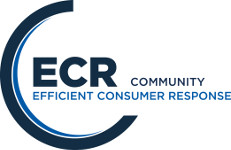
Inaccurate inventory records are a widespread challenge, particularly in retail companies: previous research carried out by the institute has shown that, on average, the items physically available in a store do not match the quantities recorded in the inventory management system for 65% of the product groups traded. These stock discrepancies impair the performance of inventory management systems, as orders can be placed too early or too late in the process. In the first case, this results in overstocking which requires unnecessary investment in inventory and can lead to space problems in the store, while in the second case, the risk of stockouts increases and therefore the availability of items in the store suffers. In many cases, retailers who want to ensure a high performance of their inventory management system only have the option of correcting data errors through expensive stock audits.
Against the background of this challenges, the Institute of Production and Supply Chain Management at TU Darmstadt (Prof. Dr. Christoph Glock) is collaborating with Cardiff Business School (Prof. Dr. Aris Syntetos) and ESCP Paris (Prof. Dr. Yacine Rekik) as well as the industry association Efficient Consumer Response (ECR) to develop machine learning methods that can be used to predict the available inventory. Initial project results show that for many product groups, the development of the actual inventory – and therefore also the data errors – can be predicted well such that inventory management can be carried out using corrected inventory data. Retail companies can then dispense with frequent stock audits for those products whose developments are easy to predict and invest the resources released in stock audits for product groups with poor predictability. In this way, resources in stock management can be focussed on those products that benefit most from stock audits in order to simultaneously save costs and increase item availability.
Once the research project is completed in December 2024, we will publish a detailed project report on this website.